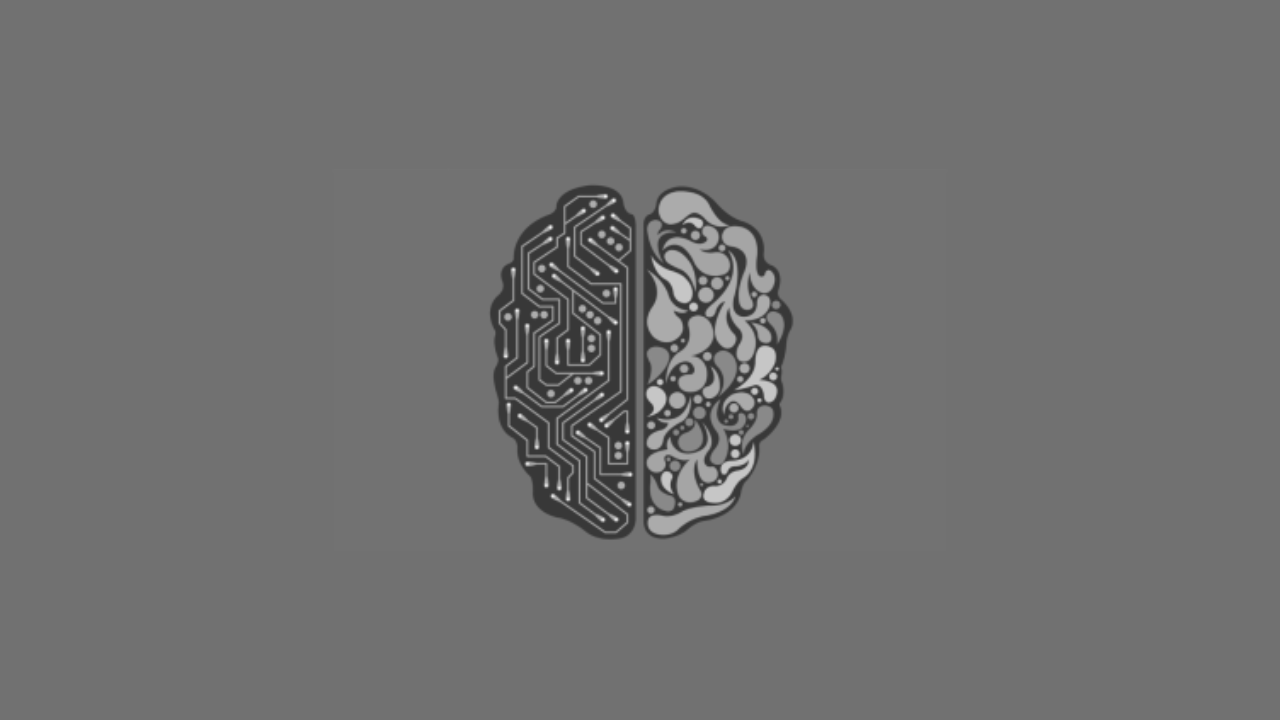
VC is a (AI-powered) Human Experience
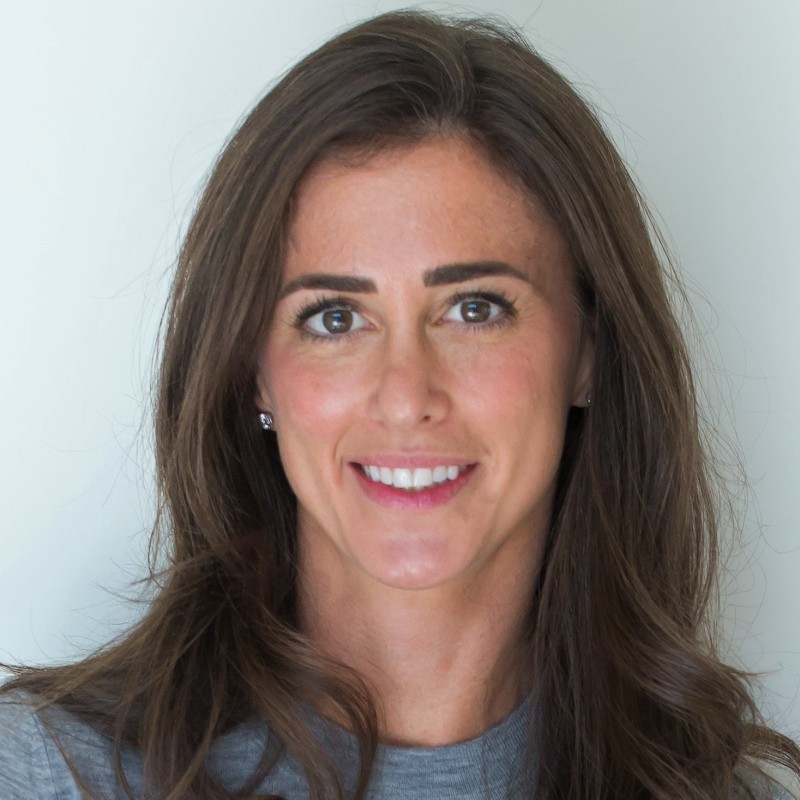
For as long as it has existed, venture capital investing has been premised on the idea that it is fundamentally a human experience – a business of relationships, networks, and partnerships. This has defined much of the VC firm’s modus operandi, from sourcing and screening all the way to due diligence and value creation.
For decades, venture capital investors have enshrined the winning formula for finding and funding successful start-ups: a strong network, plenty of intuition and… a reliable gut feeling. The formula is not flawless. The subjectivity of intuition and gut feeling, as well as the unpredictability of human behavior necessarily means that the compass could be pointing in the wrong direction.
Investors have almost accepted the margin of error. But what if they no longer had to? What happens when the formula becomes algorithmic? What if there was a technology that could match, or even surpass human intuition in recognizing the next billion-dollar start-up?
Enter artificial intelligence. While not new, the technology’s most recent manifestation is a far wider and more visible illustration of its possible use cases. At this stage of its development, AI is a non-negotiable competitive edge for all types of companies. The time to explore its business applications is here and now.
In the business of finding and funding the next tech unicorn, what does AI mean, and how can we leverage its capabilities to improve the totality of the investment process? At the same time, if AI is only as robust as its inputs, where do we draw the line between human judgment and smart machines?
Sourcing and Screening
Deal flow is a leading indicator of a venture firm’s success, and sourcing deals successfully is a factor of both volume, the quantity of deals sourced, and velocity, the speed at which the investment team screens. AI algorithms scour vast amounts of data from various sources to identify potential opportunities, solving for volume by allowing for efficient deal-sourcing. DataFox, an Oracle acquiree, aggregates multi-source intelligence of millions of public and private companies into a single platform, and uses AI to turn the data into insights that help prioritize companies, identify leads, and even predict growth trajectories.
AI then supports the screening process by analyzing key metrics and using privately gathered data on similar start-ups for benchmarking purposes. Machine learning algorithms can assess market trends, competitive landscapes, financial performance, and growth potential to provide insights into the viability and attractiveness of a start-up – all at a fraction of the speed and accuracy of the average human analyst. This data-driven approach helps investors make more informed decisions, reduces the reliance on subjective judgment and saves time when screening.
For all that it can offer at this crucial stage of the investment process, AI’s output is entirely dependent on the dataset it is pulling insights from. That data could be outdated at the very least, completely biased at most and in between, unable to keep pace with the rapidly shifting and dynamic world of technology and innovation. Considering that individuals were not necessarily ready to share pictures online or accustomed to online social activity, would an AI-powered sourcing tool have passed on Facebook back in the day?
Some billion-dollar ideas started from unprecedented and unexpected shifts in consumer behavior that AI could never have predicted or accounted for, including but not limited to sharing our homes, cars, and images of our lives.
Due Diligence
The longest and undoubtedly one of the most crucial steps of the investment process, due diligence answers the big question: do we invest?
The due diligence process is typically time-consuming, taking anywhere between a couple of weeks to a few months; data-constrained, requiring deductive analysis from sparse and fragmented databases and subjective, involving plenty of personal and/or professional judgment calls from an entire investment committee. AI can eliminate many of these pain points and elevate the due diligence process as a whole.
It goes beyond the ability to extract insights from large volumes of textual information like legal documents, contracts and financial statements. AI models can run sentiment analyses to gauge public perception of a start-up, its products and services; detect patterns and anomalies in financial data; uncover the dynamics of the competitive landscape to pinpoint advantages and disadvantages; quantify operational and market risks as well as determine the uniqueness of a company’s intellectual property assets.
AI expedites the process, while adding a layer of accuracy and capability often impossible through manual evaluation alone. Yet, in answering the “do we invest?” question, AI-based tools could still provide shortsighted or partial answers. How would AI run a sentiment or risk analysis on an early, niche or non-existent market, whether a nascent industry or an immature yet emerging geography? How would a technology that draws exclusively from quantitative datasets evaluate the soft factors that are so crucial to a startup’s success, such as team dynamics, culture and passion? On the flip-side, where human judgment is clouded by a founder’s charisma or a flawless pitch, could AI flag irregularities or improbabilities in financial projections and performance? Would it have saved investors from continuing to invest in companies like WeWork, for example? When it comes to due diligence specifically, the balance required between human intuition and technological capabilities is both intricate and delicate.
Portfolio Management
Selecting companies to invest in is only half of the work. What happens post-selection is the other, including actively managing the portfolio (through board participation and value creation), assessing risks, allocating capital for follow-on rounds and planning exits.
Arguably harder to master, portfolio management is one area of the VC engine where AI can have an outsized impact. Beyond providing a holistic view of portfolio companies with minimal manual effort through automated data aggregation, the technology can flag emerging risks, such as cash flow issues or regulatory non-compliance by monitoring financial data and overall market trends in real-time. With an average 14-year lifespan, investors are likely to invest or hold portfolio companies through at least one major global crisis. AI models prove useful in sounding early warning bells.
Other portfolio management use cases for AI include identifying areas of support for individual companies based on founder conversations, individual business performance and comparable private company data. This helps value creation efforts, allowing investors to offer tailored and targeted support to companies throughout their lifecycle, and support their success.
AI-powered predictive models can analyze historical data and market trends to estimate the optimal exit timing for portfolio companies. By considering factors such as market conditions, growth trajectory and industry dynamics, AI can provide insight on when to consider an exit strategy for maximum returns. Pitchbook’s VC Exit Predictor generates a score on the likelihood of a company to be acquired, go public or not exit using proprietary machine learning algorithms.
In the same ways that AI can support the investment process for new companies, it can also help determine whether to allocate capital for follow-on rounds into existing portfolio companies by holistically investigating the health of each individual business. In this use case, the technology’s limitations underline the need for human judgment. If one particular company is visibly slowing down but in the process of building a new product, would an AI algorithm suggest doubling down, retreating or maintaining the current position? In the case of WhatsApp, for example, would it recommend the follow-on investments, which have created significant returns for investors, or flagged an overvaluation much earlier in the company’s life, with investors missing out on the tremendous growth?
----
While the integration of AI will undoubtedly transform the landscape of venture capital, it is important to also acknowledge its consequential limitations in this context. Despite its transformative abilities, AI will not replace the power of human intuition, expertise and the value of personal connections forged in the world of investing. It is in recognizing these limitations that we truly grasp the essence of the symbiotic relationship between AI and VC.
AI serves as an exceptional supporter, complementing and amplifying the decision-making process with its analytical prowess and efficiency. Yet, in this dynamic ecosystem, it is the investor’s vision and expertise that ultimately guide the path to success.
The art of venture capital intertwines with the science of AI, creating a harmonious partnership that harnesses the best of both worlds.
Today and in the foreseeable future, VC remains a fundamentally human experience… just powered by AI.